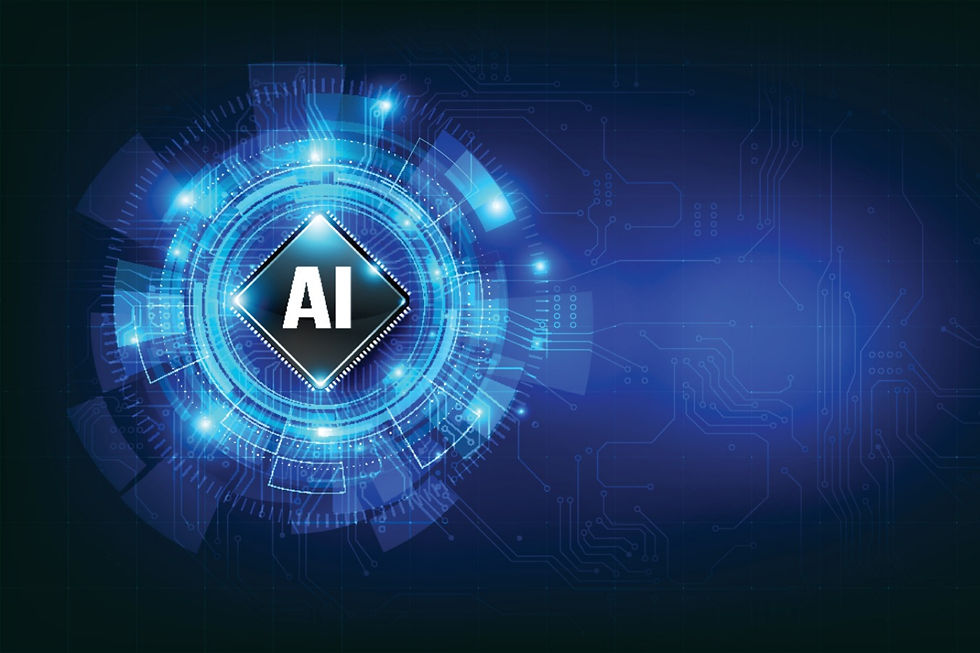
Reimagined explorer Lithium Universe will use artificial intelligence to assimilate imagery and data to assist development and optimisation of its exploration strategy at its Apollo lithium project in Canada.
The company plans to use its AI, provided by KorrAI Technologies, in concert with other geological data as part of an initial goal to fast-track exploration in its high-latitude project environment, where field-time has traditionally been restricted by the seasons.
Lithium Universe could expect a well-constructed AI system to complement and enhance the capabilities of similar and more traditional geographic information systems (GIS) by way of the machine training, learning and predictive capabilities, for which AI is noted.
It could also add a significant degree of refinement to the use of GIS, which the industry has become familiar with after using it for the past three decades. AI is likely to provide better focus and greater flexibility in data querying, pattern recognition and trend or target prediction and be of potential benefit in all applications, regardless of latitude and season.
The company restarted trading on Monday after completing a transformation from its former shell company, Mogul Games.
KorrAI has been successful at utilizing satellite data and AI technology to optimize field exploration practices in the James Bay area. They have worked for companies such as Patriot Battery Metals nearby. By employing advanced algorithms, KorrAI has created maps that identify geological features like outcrops, pegmatites, and vein formations, using spectral data to locate potential mineral deposits. This AI-driven approach enhances exploration accuracy and efficiency, allowing us to focus our field activities and resources more effectively, reducing exploration timelines and costs. Lithium Universe chairman Iggy Tan
The company has already undertaken a study on the Apollo property to explore for lithium-caesium-tantalum (LCT) mineral-bearing pegmatites using advanced technologies such as remote sensing and airborne and ground-based geophysics, combined with AI.
The study used various types of data captured by satellites and airborne systems to gather exploration information about the environment at Apollo. It included visible and infrared light, microwave signals and geomagnetic readings, which were combined to create a detailed geological picture of the exploration area.
The company used predictive AI modelling to analyse the input data and identify areas where potentially economic minerals might be present. The modelling programs were previously trained and field-tested across various sites within the James Bay region, with reference to local LCT pegmatite target types, while convolutional neural networks were used to plot predicted features of interest.
Management identified 448 priority Apollo targets and then refined them to a more manageable 28 specific areas for follow-up field sampling, mapping and ground truthing. The ground truthing was pursued through geological mapping, collection of reference field samples and photography of local topography – all keys to accurately correlating geological features, enhancing understanding for future program tweaking and reducing the incidence of false positives.
Additional datasets, including field-sample logs and analyses, high-resolution geomagnetics and hyperspectral data, would typically be used as a foundation to progressively enhance AI modelling methods. It would be expected to improve targeting accuracies over time as more relevant data was added from normal workflow.
That workflow would be comprised of the usually phased and largely unavoidable exploration process, including regional reconnaissance prospecting and mapping for geological context and identification of potential host rocks. The second phase would include evaluation of the reconnaissance results with initial target selection for more advanced stages, before a final phase that would typically include scout drilling to test prospective rocks or horizons defined by the foregoing, with the potential for a lead-in to resource drilling and definition.
Although Lithium Universe is likely to still employ the normal phases, its incorporation of AI should makes its process more focussed and efficient.
Modern-day exploration is now often based around expensive searches for deep-seated, partly or wholly-covered mineral deposits. At the same time, as expenditure gets bigger, exploration budgets often become tighter.
So while few serious advances have been made to date in the application of AI to GIS methods in mineral exploration, Lithium Universe might just prove to be an early mover in testing just how much time and expenditure can be shaved off a typical exploration program and how effectively a tricky deposit may be identified.
Is your ASX-listed company doing something interesting? Contact: office@bullsnbears.com.au